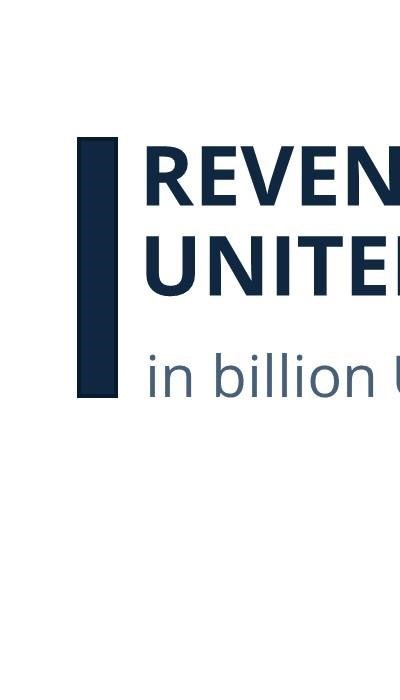
The seemingly chaotic world of dump shops and secondhand markets presents a unique opportunity for insightful data analysis. While often perceived as disorganized collections of used goods, these spaces are rich sources of data offering valuable insights for both bargain hunters and business owners. By applying techniques from data mining and retail analytics, we can uncover hidden patterns and transform seemingly random collections into opportunities for profit and understanding.
The Data Landscape of the Secondhand Market
The data available within the secondhand market is diverse and often unstructured. It includes:
- Transaction data: Sales records, including item type, price, date, and time of sale.
- Inventory data: Information on the types and quantities of goods received and available for sale.
- Customer data: While often limited, information on customer demographics and purchase history (if tracked) can be invaluable.
- External data: Market trends, competitor pricing, and seasonal factors can be incorporated for a more comprehensive analysis.
This data, however, often requires significant data cleaning before analysis. This includes handling missing values, correcting inconsistencies, and dealing with outlier analysis to identify unusual data points that might skew results. For example, an exceptionally high sale price for a common item might indicate an error or a unique buyer circumstance.
Applying Data Mining Techniques
Data mining techniques are crucial for extracting meaningful insights from the secondhand market data. These include:
Pattern Recognition and Trend Analysis
Analyzing sales data allows for pattern recognition. For instance, identifying which types of used goods sell best during specific seasons or identifying relationships between item condition and sale price. Trend analysis can reveal emerging consumer preferences, helping to inform future inventory decisions. For example, a surge in demand for vintage clothing could inform purchasing strategies.
Statistical Analysis and Market Research
Statistical analysis plays a vital role in understanding the relationships between different variables. This can inform market research efforts, allowing businesses to understand their target customer base and refine their pricing and marketing strategies. For example, regression analysis can help determine the optimal pricing strategy based on factors like item condition and demand.
Price Optimization and Inventory Management
Price optimization is crucial for maximizing profit in the competitive secondhand market. By analyzing historical sales data and market trends, businesses can dynamically adjust prices to optimize revenue. Effective inventory management, informed by sales forecasting and demand analysis, prevents overstocking of slow-moving items and ensures sufficient supply of popular items. Sales forecasting, using time series analysis, helps predict future demand and manage inventory accordingly.
Anomaly Detection and Consumer Behavior
Anomaly detection can identify unusual patterns in sales data, such as unexpected spikes or drops in demand. This can help detect potential problems, such as damaged goods, pricing errors, or shifts in consumer behavior. Understanding consumer behavior, by analyzing purchasing patterns, allows for more effective targeting and marketing strategies. For instance, identifying the preferred payment methods or shopping times of a specific demographic.
Data Visualization and Interpretation
The insights gained from data analysis are meaningless without effective communication. Data visualization tools, such as charts and graphs, are essential for presenting complex information in an easily understandable way; This allows both business owners and bargain hunters (engaging in treasure hunting) to make informed decisions.
The secondhand market, while seemingly chaotic, offers a fertile ground for data-driven insights. By employing techniques from data mining and retail analytics, businesses can optimize their operations, improve pricing strategies, and enhance their understanding of consumer behavior. For the individual bargain hunter, these same techniques can transform the experience from random treasure hunting to a more strategic and successful approach.
I found this article to be both informative and engaging. The author clearly articulates the complexities of working with secondhand market data, highlighting the importance of data cleaning and the power of various data mining techniques. The focus on practical applications, such as informing inventory decisions and understanding consumer preferences, makes the analysis relevant and accessible to a wide audience. A valuable contribution to the field.
This article provides a compelling argument for the untapped potential of data analysis within the secondhand market. The clear explanation of data sources, from transaction records to external market trends, is particularly helpful. The emphasis on data cleaning and the application of data mining techniques like pattern recognition and trend analysis demonstrates a practical understanding of the challenges and opportunities involved.
A well-structured and insightful piece. The author successfully bridges the gap between the seemingly disorganized nature of secondhand markets and the potential for rigorous data analysis. The examples provided, such as identifying seasonal trends and analyzing the relationship between item condition and price, effectively illustrate the practical applications of the discussed techniques. This article would be valuable to both researchers and business professionals interested in this field.